Multisource data fusion for enhanced gold mineral prospectivity mapping in Yagba West, Kogi State: a machine learning approach
Keywords:
Gold mineral, Support vector machine, Classification and regression trees, Yagba westAbstract
Gold mineral prospectivity mapping is crucial for identifying potential gold-bearing zones and supporting exploration efforts through advanced data analytics. However, many existing models tend to overestimate high-prospectivity areas, introducing biases toward known deposits and limiting their effectiveness in discovering new mineralized zones. To enhance exploration accuracy, data-driven approaches that enhance model interpretability and minimize predictive bias are essential. In this study, we applied Support Vector Machines (SVM) and Classification and Regression Trees (CART) to generate gold mineralization maps for Yagba West, utilizing an integrated dataset comprising SRTM DEM, Landsat 8 imagery, geological maps, and aeromagnetic data. The SVM model identified a gold-prospective area of 249.58 km² with an accuracy of 100%, while the CART model delineated a 132.13 km² prospective zone with an accuracy of 93%. This study further revealed that gold occurrences in the study area are predominantly concentrated in quartzite, quartz schist, gabbro, and quartz gabbro formations, primarily along NNE–SSW and NW–SE structural orientations, emphasizing the influence of structural controls on mineralization. These findings underscore the potential of machine learning in enhancing gold prospectivity mapping and optimizing exploration strategies in structurally controlled gold-bearing terrains.
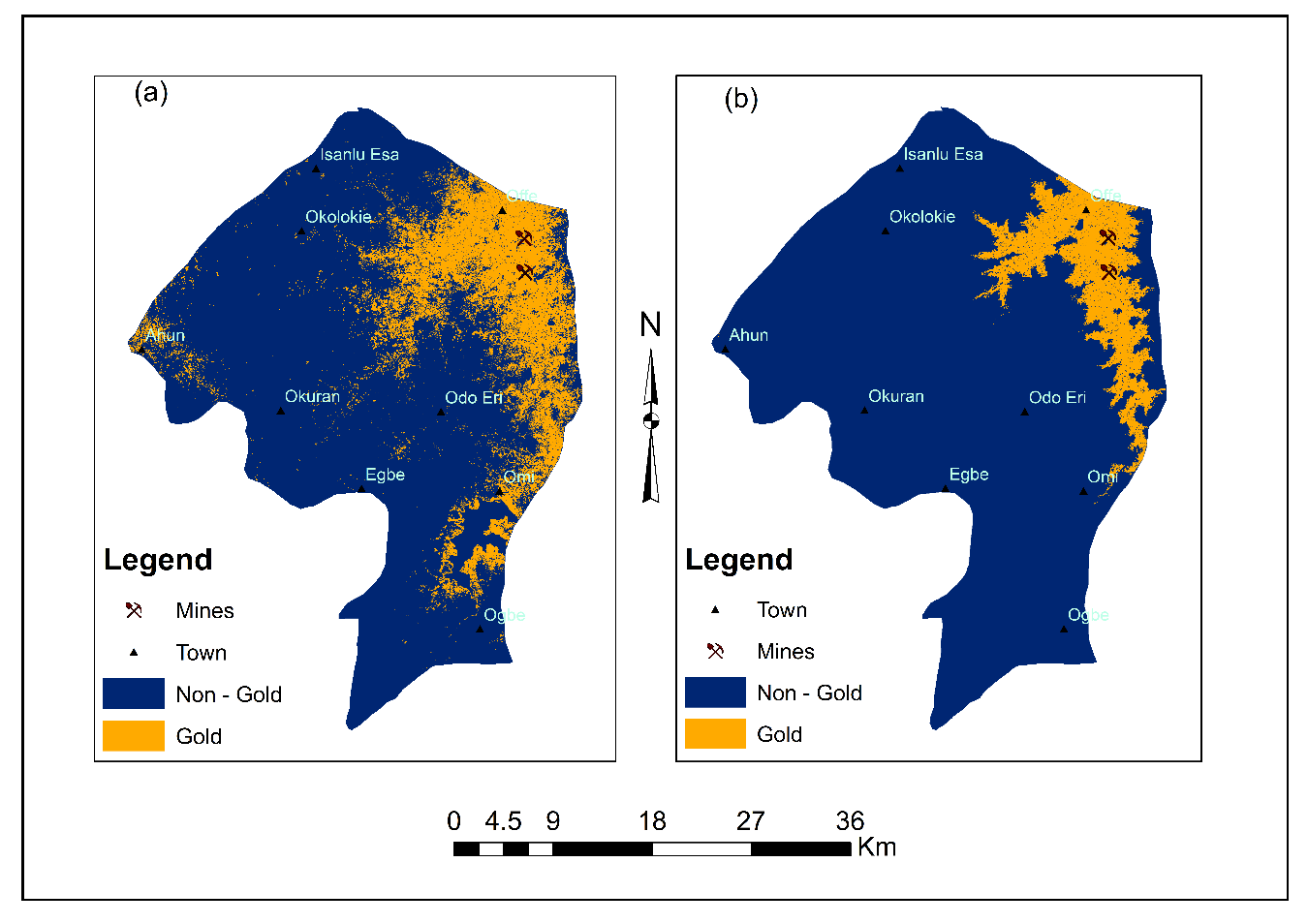
Published
How to Cite
Issue
Section
Copyright (c) 2025 Momohjimoh Abdulsalami, Jacob Funsho Omonile, Abubakar Fahad, Abdullateef Aliyu, Muhammad Kabir Yahaya (Author)

This work is licensed under a Creative Commons Attribution 4.0 International License.