Stacking ensemble machine learning for predicting land surface temperature hotspots using landsat 9 data
Keywords:
LST, Geothermal energy, Machine learning, Landsat 9Abstract
Despite advancements in predictive modeling, existing methods struggle with accuracy and spatial variability in Land Surface Temperature (LST) estimation. This study presents a Stacking Ensemble Model (SEM) integrating Random Forest (RF), eXtreme Gradient Boosting (XGBoost), and k-Nearest Neighbors (KNN) to enhance LST prediction using Landsat 9 and SRTM DEM data in Kogi State, Nigeria. The SEM outperformed individual models, achieving an R² of 99.86%, surpassing RF by 3.31%, XGBoost by 8.03%, and KNN by 12.79%. Results revealed significant spatial variability, with temperatures ranging from 24.8°C to 49.3°C and critical hotspots above 40°C covering 1,035 km², supporting geothermal energy exploration. Incorporating elevation spectral indices and key predictors like NDVI, proportion of vegetation, land surface emissivity, and brightness temperature further improved accuracy. This SEM framework enhances predictive robustness, scalability, and spatial analysis for better LST modeling.
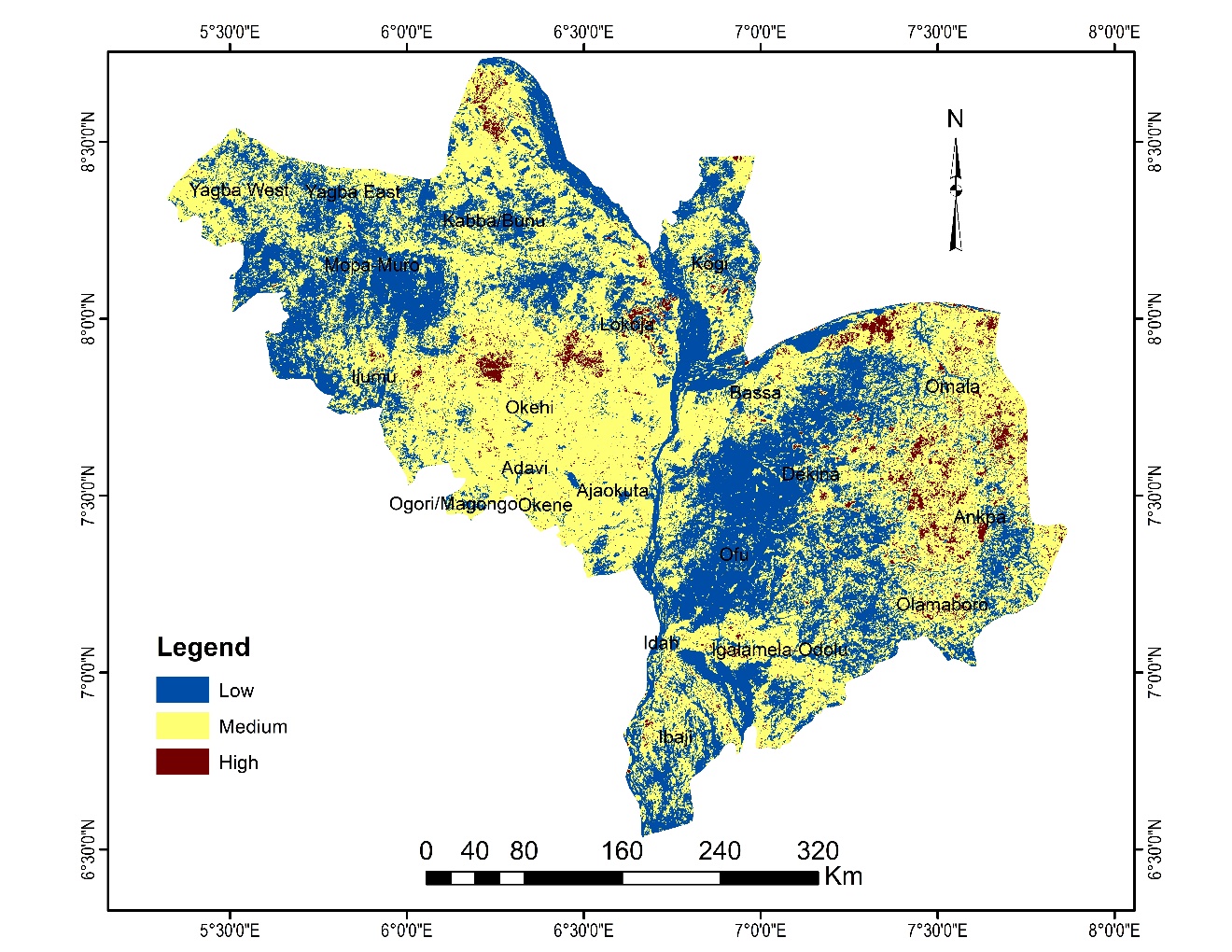
Published
How to Cite
Issue
Section
Copyright (c) 2025 Momohjimoh Abdulsalami, Dahiru Dahuwa, Saratu Muhammad Hussaini, Yahaya Jibrin Danjuma, Michael Adewale Ibitomi, Danga Onimisi Abdulmalik, Bunmi Oyekola Isaac, Zainab Usman, Joseph Omeiza Alao, Aliyu Abdullateef (Author)

This work is licensed under a Creative Commons Attribution 4.0 International License.