Modelling patients waiting and service time by ARIMA model: a case of Federal University Gusau clinic
Keywords:
Waiting Time, Service Time, ARIMA, Modelling, OutliersAbstract
The ability to model and forecast waiting and service time to increase patients' satisfaction, reduce waiting time, avoid casualties, and increase efficiency in service delivery is crucial. It encourages the identification of future pressure by using the relevant key performance indicators. In this paper, the ARIMA model is used to study the waiting and service time of patients at the {\it Federal University Gusau} Health Services Clinic. The system was a single, time-independent arrival with many service points. Based on the results found in the waiting and service processes, the service time has a lower mean and variance when compared to the waiting time. The waiting time has a lower skewness and kurtosis when compared to the service time. The Ljung-Box (Q) Statistic test shows that the correlation in the time series has been adequately captured for the waiting and service time processes, though the waiting and service time processes have 4 and 10 outliers respectively. The ARIMA (0,1,2) and ARIMA (2,1,1) are selected for modelling the waiting and service time respectively based on the evaluation metrics.
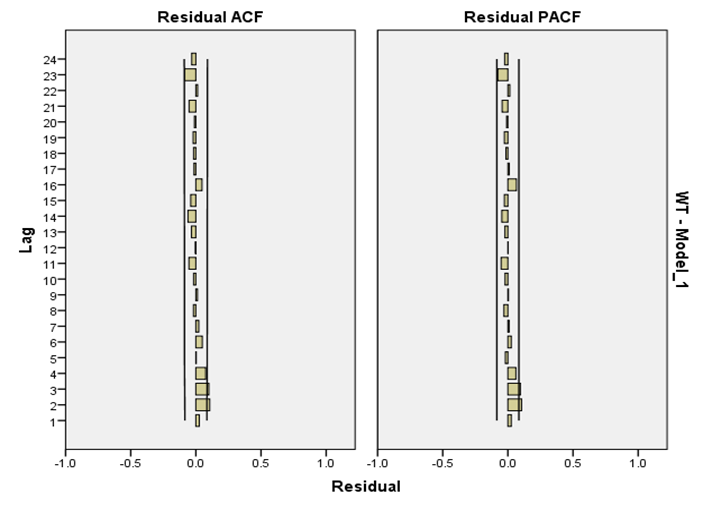
Published
How to Cite
Issue
Section
Copyright (c) 2023 Aliyu Usman Moyi, Kabir Bello, Olayemi Joshua Ibidoja, Garba Muhammad

This work is licensed under a Creative Commons Attribution 4.0 International License.