Global convergence properties of a Dai-Liao-type CGM for unconstrained optimization
Keywords:
Unconstrained optimization, Convergence properties, Descent directions, Objective functionAbstract
A popular optimization technique called the conjugate gradient method (CGM) is renowned for its effectiveness in addressing problems involving unconstrained optimization. Several conjugate gradient (CG) techniques have been proven to possess global convergence properties both theoretically and numerically. The Dai-Liao-type CGM is a variant that incorporates certain modifications to enhance its convergence properties. This paper examines the global convergence properties of a Dai-Liao-type CGM for unconstrained optimization problems. Theoretically, this study investigates the conditions under which the method ensures convergence to the global minimum of the objective function, focusing on the algorithm’s descent directions, the necessary reduction in objective function values, and termination criteria. A numerical experiment is conducted on a set of unconstrained optimization problems to validate the theoretical results obtained in this work. The numerical findings of this study demonstrate the robustness and reliability of the Dai-Liao-type CGM, showing its ability to find the global optimal solution in a wide range of unconstrained optimization problems.
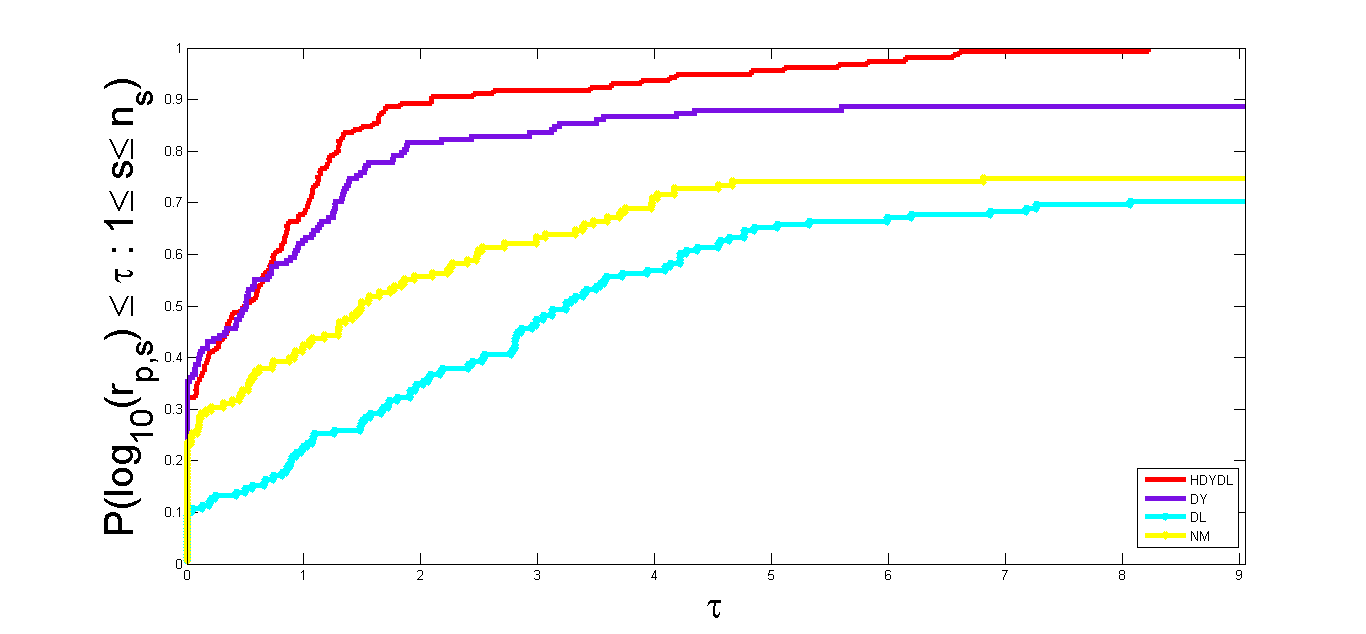
Published
How to Cite
Issue
Section
Copyright (c) 2024 Oluwaseun Biodun Onuoha

This work is licensed under a Creative Commons Attribution 4.0 International License.